AI+无线通信总结——初赛算法实现(Top37)
【摘要】 先上代码。剩下的有时间在补,最终提交的初赛排名是37,但是没有跑完,跑完应该能进前20,但是由于算力不足,只跑了50个epoch。我先抛个砖,希望能引来玉。完整代码:Model_define_pytorch.py#!/usr/bin/env python3import numpy as npimport torch.nn as nnimport torchimport torch.nn....
先上代码。剩下的有时间在补,最终提交的初赛排名是37,但是没有跑完,跑完应该能进前20,但是由于算力不足,只跑了50个epoch。我先抛个砖,希望能引来玉。
完整代码:
Model_define_pytorch.py
#!/usr/bin/env python3
import numpy as np
import torch.nn as nn
import torch
import torch.nn.functional as F
from torch.utils.data import Dataset
from collections import OrderedDict
channelNum = 64
class Mish(torch.nn.Module):
def __init__(self):
super().__init__()
def forward(self, x):
x = x * (torch.tanh(torch.nn.functional.softplus(x)))
return x
# This part implement the quantization and dequantization operations.
# The output of the encoder must be the bitstream.
def Num2Bit(Num, B):
Num_ = Num.type(torch.uint8)
def integer2bit(integer, num_bits=B * 2):
dtype = integer.type()
exponent_bits = -torch.arange(-(num_bits - 1), 1).type(dtype)
exponent_bits = exponent_bits.repeat(integer.shape + (1,))
out = integer.unsqueeze(-1) // 2 ** exponent_bits
return (out - (out % 1)) % 2
bit = integer2bit(Num_)
bit = (bit[:, :, B:]).reshape(-1, Num_.shape[1] * B)
return bit.type(torch.float32)
def Bit2Num(Bit, B):
Bit_ = Bit.type(torch.float32)
Bit_ = torch.reshape(Bit_, [-1, int(Bit_.shape[1] / B), B])
num = torch.zeros(Bit_[:, :, 1].shape).cuda()
for i in range(B):
num = num + Bit_[:, :, i] * 2 ** (B - 1 - i)
return num
class Quantization(torch.autograd.Function):
@staticmethod
def forward(ctx, x, B):
ctx.constant = B
step = 2 ** B
out = torch.round(x * step - 0.5)
out = Num2Bit(out, B)
return out
@staticmethod
def backward(ctx, grad_output):
# return as many input gradients as there were arguments.
# Gradients of constant arguments to forward must be None.
# Gradient of a number is the sum of its four bits.
b, _ = grad_output.shape
grad_num = torch.sum(grad_output.reshape(b, -1, ctx.constant), dim=2)
return grad_num, None
class Dequantization(torch.autograd.Function):
@staticmethod
def forward(ctx, x, B):
ctx.constant = B
step = 2 ** B
out = Bit2Num(x, B)
out = (out + 0.5) / step
return out
@staticmethod
def backward(ctx, grad_output):
# return as many input gradients as there were arguments.
# Gradients of non-Tensor arguments to forward must be None.
# repeat the gradient of a Num for B time.
b, c = grad_output.shape
grad_output = grad_output.unsqueeze(2) / ctx.constant
grad_bit = grad_output.expand(b, c, ctx.constant)
return torch.reshape(grad_bit, (-1, c * ctx.constant)), None
class QuantizationLayer(nn.Module):
def __init__(self, B):
super(QuantizationLayer, self).__init__()
self.B = B
def forward(self, x):
out = Quantization.apply(x, self.B)
return out
class ResBlock(nn.Module):
"""
Sequential residual blocks each of which consists of \
two convolution layers.
Args:
ch (int): number of input and output channels.
nblocks (int): number of residual blocks.
shortcut (bool): if True, residual tensor addition is enabled.
"""
def __init__(self, ch, nblocks=1, shortcut=True):
super().__init__()
self.shortcut = shortcut
self.module_list = nn.ModuleList()
for i in range(nblocks):
resblock_one = nn.ModuleList()
resblock_one.append(ConvBN(ch, ch, 1))
resblock_one.append(Mish())
resblock_one.append(ConvBN(ch, ch, 3))
resblock_one.append(Mish())
self.module_list.append(resblock_one)
def forward(self, x):
for module in self.module_list:
h = x
for res in module:
h = res(h)
x = x + h if self.shortcut else h
return x
class DequantizationLayer(nn.Module):
def __init__(self, B):
super(DequantizationLayer, self).__init__()
self.B = B
def forward(self, x):
out = Dequantization.apply(x, self.B)
return out
def conv3x3(in_planes, out_planes, stride=1):
"""3x3 convolution with padding"""
return nn.Conv2d(in_planes, out_planes, kernel_size=3, stride=stride,
padding=1, bias=True)
class ConvBN(nn.Sequential):
def __init__(self, in_planes, out_planes, kernel_size, stride=1, groups=1):
if not isinstance(kernel_size, int):
padding = [(i - 1) // 2 for i in kernel_size]
else:
padding = (kernel_size - 1) // 2
super(ConvBN, self).__init__(OrderedDict([
('conv', nn.Conv2d(in_planes, out_planes, kernel_size, stride,
padding=padding, groups=groups, bias=False)),
('bn', nn.BatchNorm2d(out_planes)),
('Mish', Mish())
]))
class CRBlock64(nn.Module):
def __init__(self):
super(CRBlock64, self).__init__()
self.convbncrb = ConvBN(channelNum, channelNum * 2, 3)
self.path1 = Encoder_conv(channelNum * 2, 4)
self.path2 = nn.Sequential(OrderedDict([
('conv1x5', ConvBN(channelNum * 2, channelNum * 2, [1, 5])),
('conv5x1', ConvBN(channelNum * 2, channelNum * 2, [5, 1])),
('conv5x1', ConvBN(channelNum * 2, channelNum * 2, 1)),
('conv5x1', ConvBN(channelNum * 2, channelNum * 2, 3)),
]))
self.encoder_conv = Encoder_conv(channelNum * 4, 4)
self.encoder_conv1 = ConvBN(channelNum * 4, channelNum, 1)
self.identity = nn.Identity()
self.relu = Mish()
def forward(self, x):
identity = self.identity(x)
x = self.convbncrb(x)
out1 = self.path1(x)
out2 = self.path2(x)
out = torch.cat((out1, out2), dim=1)
out = self.relu(out)
out = self.encoder_conv(out)
out = self.encoder_conv1(out)
out = self.relu(out + identity)
return out
class CRBlock(nn.Module):
def __init__(self):
super(CRBlock, self).__init__()
self.convban = nn.Sequential(OrderedDict([
("conv3x3_bn", ConvBN(channelNum, channelNum, 3)),
]))
self.path1 = Encoder_conv(channelNum, 4)
self.path2 = nn.Sequential(OrderedDict([
('conv1x5', ConvBN(channelNum, channelNum, [1, 5])),
('conv5x1', ConvBN(channelNum, channelNum, [5, 1])),
("conv9x1_bn", ConvBN(channelNum, channelNum, 1)),
]))
self.encoder_conv = Encoder_conv(channelNum * 2)
self.encoder_conv1 = ConvBN(channelNum * 2, channelNum, 1)
self.identity = nn.Identity()
self.relu = Mish()
def forward(self, x):
identity = self.identity(x)
x = self.convban(x)
out1 = self.path1(x)
out2 = self.path2(x)
out = torch.cat((out1, out2), dim=1)
out = self.relu(out)
out = self.encoder_conv(out)
out = self.encoder_conv1(out)
out = self.relu(out + identity)
return out
class Encoder_conv(nn.Module):
def __init__(self, in_planes=128, blocks=2):
super().__init__()
self.conv2 = ConvBN(in_planes, in_planes, [1, 9])
self.conv3 = ConvBN(in_planes, in_planes, [9, 1])
self.conv4 = ConvBN(in_planes, in_planes, 1)
self.resBlock = ResBlock(ch=in_planes, nblocks=blocks)
self.conv5 = ConvBN(in_planes, in_planes, [1, 7])
self.conv6 = ConvBN(in_planes, in_planes, [7, 1])
self.conv7 = ConvBN(in_planes, in_planes, 1)
self.relu = Mish()
def forward(self, input):
x2 = self.conv2(input)
x3 = self.conv3(x2)
x4 = self.conv4(x3)
r1 = self.resBlock(x4)
x5 = self.conv5(r1)
x6 = self.conv6(x5)
x7 = self.conv7(x6)
x7 = self.relu(x7 + x4)
return x7
class Encoder(nn.Module):
B = 4
def __init__(self, feedback_bits, quantization=True):
super(Encoder, self).__init__()
self.convban = nn.Sequential(OrderedDict([
("conv3x3_bn", ConvBN(2, channelNum, 3)),
]))
self.encoder1 = Encoder_conv(channelNum)
self.encoder2 = nn.Sequential(OrderedDict([
('conv1x5', ConvBN(channelNum, channelNum, [1, 5])),
('conv5x1', ConvBN(channelNum, channelNum, [5, 1])),
("conv9x1_bn", ConvBN(channelNum, channelNum, 3)),
]))
self.encoder_conv = Encoder_conv(channelNum * 2)
self.encoder_conv1 = nn.Sequential(OrderedDict([
("conv1x1_bn", ConvBN(channelNum * 2, 2, 1)),
]))
self.fc = nn.Linear(1024, int(feedback_bits / self.B))
self.sig = nn.Sigmoid()
self.quantize = QuantizationLayer(self.B)
self.quantization = quantization
def forward(self, x):
x = self.convban(x)
encode1 = self.encoder1(x)
encode2 = self.encoder2(x)
out = torch.cat((encode1, encode2), dim=1)
out = self.encoder_conv(out)
out = self.encoder_conv1(out)
out = out.view(-1, 1024)
out = self.fc(out)
out = self.sig(out)
if self.quantization:
out = self.quantize(out)
else:
out = out
return out
class Decoder(nn.Module):
B = 4
def __init__(self, feedback_bits, quantization=True):
super(Decoder, self).__init__()
self.feedback_bits = feedback_bits
self.dequantize = DequantizationLayer(self.B)
self.fc = nn.Linear(int(feedback_bits / self.B), 1024)
decoder = OrderedDict([
("conv3x3_bn", ConvBN(2, channelNum, 3)),
("CRBlock1", CRBlock64()),
("CRBlock2", CRBlock()),
])
self.decoder_feature = nn.Sequential(decoder)
self.out_cov = conv3x3(channelNum, 2)
self.sig = nn.Sigmoid()
self.quantization = quantization
def forward(self, x):
if self.quantization:
out = self.dequantize(x)
else:
out = x
out = out.view(-1, int(self.feedback_bits / self.B))
out = self.fc(out)
out = out.view(-1, 2, 16, 32)
out = self.decoder_feature(out)
out = self.out_cov(out)
out = self.sig(out)
return out
# Note: Do not modify following class and keep it in your submission.
# feedback_bits is 128 by default.
class AutoEncoder(nn.Module):
def __init__(self, feedback_bits):
super(AutoEncoder, self).__init__()
self.encoder = Encoder(feedback_bits)
self.decoder = Decoder(feedback_bits)
def forward(self, x):
feature = self.encoder(x)
out = self.decoder(feature)
return out
def NMSE(x, x_hat):
x_real = np.reshape(x[:, :, :, 0], (len(x), -1))
x_imag = np.reshape(x[:, :, :, 1], (len(x), -1))
x_hat_real = np.reshape(x_hat[:, :, :, 0], (len(x_hat), -1))
x_hat_imag = np.reshape(x_hat[:, :, :, 1], (len(x_hat), -1))
x_C = x_real - 0.5 + 1j * (x_imag - 0.5)
x_hat_C = x_hat_real - 0.5 + 1j * (x_hat_imag - 0.5)
power = np.sum(abs(x_C) ** 2, axis=1)
mse = np.sum(abs(x_C - x_hat_C) ** 2, axis=1)
nmse = np.mean(mse / power)
return nmse
def NMSE_cuda(x, x_hat):
x_real = x[:, 0, :, :].view(len(x), -1) - 0.5
x_imag = x[:, 1, :, :].view(len(x), -1) - 0.5
x_hat_real = x_hat[:, 0, :, :].view(len(x_hat), -1) - 0.5
x_hat_imag = x_hat[:, 1, :, :].view(len(x_hat), -1) - 0.5
power = torch.sum(x_real ** 2 + x_imag ** 2, axis=1)
mse = torch.sum((x_real - x_hat_real) ** 2 + (x_imag - x_hat_imag) ** 2, axis=1)
nmse = mse / power
return nmse
class NMSELoss(nn.Module):
def __init__(self, reduction='sum'):
super(NMSELoss, self).__init__()
self.reduction = reduction
def forward(self, x_hat, x):
nmse = NMSE_cuda(x, x_hat)
if self.reduction == 'mean':
nmse = torch.mean(nmse)
else:
nmse = torch.sum(nmse)
return nmse
def Score(NMSE):
score = 1 - NMSE
return score
# dataLoader
class DatasetFolder(Dataset):
def __init__(self, matData):
self.matdata = matData
def __len__(self):
return self.matdata.shape[0]
def __getitem__(self, index):
return self.matdata[index] # , self.matdata[index]

Model_train.py
'''
seefun . Aug 2020.
github.com/seefun | kaggle.com/seefun
'''
import numpy as np
import h5py
import torch
import os
import torch.nn as nn
import random
from Model_define_pytorch import AutoEncoder, DatasetFolder, NMSE_cuda, NMSELoss
# Parameters for training
gpu_list = '0'
os.environ["CUDA_VISIBLE_DEVICES"] = gpu_list
def seed_everything(seed=42):
random.seed(seed)
os.environ['PYHTONHASHSEED'] = str(seed)
np.random.seed(seed)
torch.manual_seed(seed)
torch.cuda.manual_seed(seed)
torch.backends.cudnn.deterministic = True
SEED = 42
seed_everything(SEED)
batch_size = 32
epochs = 150
learning_rate = 1e-3 # bigger to train faster
num_workers = 0
print_freq = 100
train_test_ratio = 0.8
# parameters for data
feedback_bits = 128
img_height = 16
img_width = 32
img_channels = 2
# Model construction
model = AutoEncoder(feedback_bits)
model.encoder.quantization = False
model.decoder.quantization = False
if len(gpu_list.split(',')) > 1:
model = torch.nn.DataParallel(model).cuda() # model.module
else:
model = model.cuda()
criterion = NMSELoss(reduction='mean') # nn.MSELoss()
criterion_test = NMSELoss(reduction='sum')
optimizer = torch.optim.AdamW(model.parameters(), lr=learning_rate)
# Data loading
data_load_address = './data'
mat = h5py.File(data_load_address + '/Hdata.mat', 'r')
data = np.transpose(mat['H_train']) # shape=(320000, 1024)
data = data.astype('float32')
data = np.reshape(data, [len(data), img_channels, img_height, img_width])
# split data for training(80%) and validation(20%)
np.random.shuffle(data)
start = int(data.shape[0] * train_test_ratio)
x_train, x_test = data[:start], data[start:]
# dataLoader for training
train_dataset = DatasetFolder(x_train)
train_loader = torch.utils.data.DataLoader(
train_dataset, batch_size=batch_size, shuffle=True, num_workers=num_workers, pin_memory=True, drop_last=True)
# dataLoader for training
test_dataset = DatasetFolder(x_test)
test_loader = torch.utils.data.DataLoader(
test_dataset, batch_size=batch_size, shuffle=False, num_workers=num_workers, pin_memory=True)
best_loss = 100
for epoch in range(epochs):
print('========================')
print('lr:%.4e' % optimizer.param_groups[0]['lr'])
# model training
model.train()
if epoch < epochs // 10:
try:
model.encoder.quantization = False
model.decoder.quantization = False
except:
model.module.encoder.quantization = False
model.module.decoder.quantization = False
else:
try:
model.encoder.quantization = True
model.decoder.quantization = True
except:
model.module.encoder.quantization = True
model.module.decoder.quantization = True
if epoch % 50 == 0 and epoch > 0:
optimizer.param_groups[0]['lr'] = optimizer.param_groups[0]['lr'] * 0.1
for i, input in enumerate(train_loader):
input = input.cuda()
output = model(input)
loss = criterion(output, input)
loss.backward()
optimizer.step()
optimizer.zero_grad()
if i % print_freq == 0:
print('Epoch: [{0}][{1}/{2}]\t'
'Loss {loss:.4f}\t'.format(
epoch, i, len(train_loader), loss=loss.item()))
model.eval()
try:
model.encoder.quantization = True
model.decoder.quantization = True
except:
model.module.encoder.quantization = True
model.module.decoder.quantization = True
total_loss = 0
with torch.no_grad():
for i, input in enumerate(test_loader):
# convert numpy to Tensor
input = input.cuda()
output = model(input)
total_loss += criterion_test(output, input).item()
average_loss = total_loss / len(test_dataset)
print('NMSE %.4f' % average_loss)
if average_loss < best_loss:
# model save
# save encoder
modelSave1 = './Modelsave/encoder.pth.tar'
try:
torch.save({'state_dict': model.encoder.state_dict(), }, modelSave1)
except:
torch.save({'state_dict': model.module.encoder.state_dict(), }, modelSave1)
# save decoder
modelSave2 = './Modelsave/decoder.pth.tar'
try:
torch.save({'state_dict': model.decoder.state_dict(), }, modelSave2)
except:
torch.save({'state_dict': model.module.decoder.state_dict(), }, modelSave2)
print('Model saved!')
best_loss = average_loss

推荐
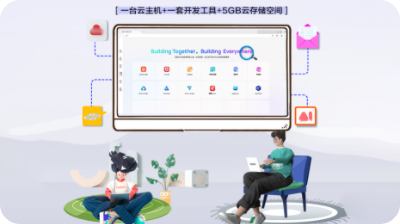
华为开发者空间发布
让每位开发者拥有一台云主机
【版权声明】本文为华为云社区用户原创内容,转载时必须标注文章的来源(华为云社区)、文章链接、文章作者等基本信息, 否则作者和本社区有权追究责任。如果您发现本社区中有涉嫌抄袭的内容,欢迎发送邮件进行举报,并提供相关证据,一经查实,本社区将立刻删除涉嫌侵权内容,举报邮箱:
cloudbbs@huaweicloud.com
- 点赞
- 收藏
- 关注作者
评论(0)